This is a continuation of the previous post. Here I will apply the Black Litterman model to a portfolio of stocks.
import pandas as pd
import yfinance as yf
import openpyxl
from currency_converter import CurrencyConverter
import numpy as np
from pypfopt import BlackLittermanModel
from pypfopt import black_litterman, risk_models
# Declare constants
PORTFOLIO='/mnt/wd-bigfoot/share/luis/OneDrive/Documents/Portfolio/00 portfolio.xlsx'
STOCKS_SHEET='Stocks'
START_DATE='2010-01-01'
END_DATE='2021-01-08'
ALL_WORLD='IWDA.AS'
TOTAL_FUNDS=40e3 # 40.000 EUR
# Open the portfolio and get the stocks
stocks_pd = pd.read_excel(PORTFOLIO, engine='openpyxl', sheet_name=STOCKS_SHEET)
stocks_pd.index = stocks_pd['Symbol']
stocks_pd = stocks_pd[['Company Name', 'Prediction', 'Pesimistic', 'Optimistic']]
stocks_pd
|
Company Name |
Prediction |
Pesimistic |
Optimistic |
Symbol |
|
|
|
|
AMD |
Advanced Micro Devices, Inc. |
0.20 |
0.03 |
0.40 |
AIR.PA |
Airbus SE |
0.10 |
0.03 |
0.20 |
GOOGL |
Alphabet Inc. |
0.10 |
0.03 |
0.30 |
AMZN |
Amazon.com, Inc. |
0.20 |
0.05 |
0.50 |
AAPL |
Apple Inc. |
0.15 |
0.00 |
0.40 |
BA.L |
BAE Systems plc |
0.00 |
-0.05 |
0.05 |
CSCO |
Cisco Systems, Inc. |
0.00 |
-0.05 |
0.05 |
BN.PA |
Danone S.A. |
0.00 |
-0.05 |
0.05 |
FB |
Facebook, Inc. |
0.05 |
-0.05 |
0.05 |
GE |
General Electric Company |
0.05 |
0.00 |
0.05 |
HYU.F |
Hyundai Corporation |
0.10 |
0.00 |
0.30 |
IDR.MC |
Indra Sistemas, S.A. |
0.00 |
-0.05 |
0.02 |
INTC |
Intel Corporation |
0.00 |
-0.02 |
0.05 |
IBM |
International Business Machines Corporation |
0.05 |
-0.02 |
0.10 |
IAG.MC |
International Consolidated Airlines Group, S.A. |
0.50 |
-0.10 |
1.00 |
PHIA.AS |
Koninklijke Philips N.V. |
0.00 |
-0.05 |
0.05 |
MEL.MC |
Meliá Hotels International, S.A. |
0.20 |
0.00 |
0.50 |
MRK |
Merck & Co., Inc. |
0.05 |
0.00 |
0.05 |
MSFT |
Microsoft Corporation |
0.10 |
0.00 |
0.15 |
NESN.SW |
Nestlé S.A. |
0.00 |
-0.05 |
0.03 |
NFLX |
Netflix, Inc. |
0.05 |
-0.01 |
0.15 |
NHH.MC |
NH Hotel Group, S.A. |
0.20 |
-0.05 |
0.50 |
NOKIA.HE |
Nokia Corporation |
0.05 |
-0.05 |
0.20 |
NVDA |
NVIDIA Corporation |
0.10 |
-0.05 |
0.30 |
ORCL |
Oracle Corporation |
0.05 |
0.00 |
0.05 |
PEP |
PepsiCo, Inc. |
0.00 |
-0.05 |
0.05 |
QCOM |
QUALCOMM Incorporated |
0.05 |
-0.02 |
0.15 |
SSUN.F |
Samsung Electronics Co., Ltd. |
0.10 |
-0.02 |
0.20 |
SIE.DE |
Siemens Aktiengesellschaft |
0.05 |
-0.02 |
0.10 |
ENR.DE |
Siemens Energy AG |
0.05 |
-0.02 |
0.10 |
SPOT |
Spotify Technology S.A. |
0.02 |
-0.05 |
0.10 |
TSLA |
Tesla, Inc. |
0.00 |
-0.20 |
0.05 |
TXN |
Texas Instruments Incorporated |
0.05 |
0.00 |
0.10 |
BA |
The Boeing Company |
0.05 |
-0.05 |
0.10 |
KHC |
The Kraft Heinz Company |
0.00 |
-0.05 |
0.05 |
PG |
The Procter & Gamble Company |
0.05 |
-0.05 |
0.05 |
DIS |
The Walt Disney Company |
0.05 |
-0.05 |
0.20 |
TMO |
Thermo Fisher Scientific Inc. |
0.05 |
-0.05 |
0.15 |
TM |
Toyota Motor Corporation |
0.05 |
-0.02 |
0.10 |
UBER |
Uber Technologies, Inc. |
0.10 |
-0.10 |
0.20 |
UL |
Unilever PLC |
0.00 |
-0.05 |
0.05 |
# Get the historical data of the stocks and exchange to EUR
stocks = pd.DataFrame()
cc = CurrencyConverter(fallback_on_missing_rate=True, fallback_on_wrong_date='True')
for symbol in stocks_pd.index:
history = yf.download(symbol, start=START_DATE, end=END_DATE)['Adj Close']
dataframe = pd.DataFrame(history)
currency = yf.Ticker(symbol).info['currency'].upper()
dataframe.columns = [ symbol + ':' + currency ]
# Add Market Cap to the stocks_pd
stocks_pd.at[symbol, 'Market Cap'] = cc.convert(yf.Ticker(symbol).info['marketCap'],
currency, 'EUR',
dataframe.index[-1].to_pydatetime())
# Add column with security in EUR if needed
if currency.lower() != 'eur':
for index in dataframe.index:
original_value = dataframe.at[index, symbol + ':' + currency]
value = cc.convert(original_value, currency, 'EUR', date=index.to_pydatetime())
dataframe.at[index, symbol + ':EUR'] = value
# Merge everything
stocks = pd.concat([ stocks, dataframe], axis='columns')
# Fill any NA values with the best forward or backarwd value
stocks.fillna(method='ffill', inplace=True)
del cc, symbol, history, dataframe, currency, original_value, value, index
[*********************100%***********************] 1 of 1 completed
[*********************100%***********************] 1 of 1 completed
[*********************100%***********************] 1 of 1 completed
[*********************100%***********************] 1 of 1 completed
[*********************100%***********************] 1 of 1 completed
[*********************100%***********************] 1 of 1 completed
[*********************100%***********************] 1 of 1 completed
[*********************100%***********************] 1 of 1 completed
[*********************100%***********************] 1 of 1 completed
[*********************100%***********************] 1 of 1 completed
[*********************100%***********************] 1 of 1 completed
[*********************100%***********************] 1 of 1 completed
[*********************100%***********************] 1 of 1 completed
[*********************100%***********************] 1 of 1 completed
[*********************100%***********************] 1 of 1 completed
[*********************100%***********************] 1 of 1 completed
[*********************100%***********************] 1 of 1 completed
[*********************100%***********************] 1 of 1 completed
[*********************100%***********************] 1 of 1 completed
[*********************100%***********************] 1 of 1 completed
[*********************100%***********************] 1 of 1 completed
[*********************100%***********************] 1 of 1 completed
[*********************100%***********************] 1 of 1 completed
[*********************100%***********************] 1 of 1 completed
[*********************100%***********************] 1 of 1 completed
[*********************100%***********************] 1 of 1 completed
[*********************100%***********************] 1 of 1 completed
[*********************100%***********************] 1 of 1 completed
[*********************100%***********************] 1 of 1 completed
[*********************100%***********************] 1 of 1 completed
[*********************100%***********************] 1 of 1 completed
[*********************100%***********************] 1 of 1 completed
[*********************100%***********************] 1 of 1 completed
[*********************100%***********************] 1 of 1 completed
[*********************100%***********************] 1 of 1 completed
[*********************100%***********************] 1 of 1 completed
[*********************100%***********************] 1 of 1 completed
[*********************100%***********************] 1 of 1 completed
[*********************100%***********************] 1 of 1 completed
[*********************100%***********************] 1 of 1 completed
[*********************100%***********************] 1 of 1 completed
|
Company Name |
Prediction |
Pesimistic |
Optimistic |
Market Cap |
Symbol |
|
|
|
|
|
AMD |
Advanced Micro Devices, Inc. |
0.20 |
0.03 |
0.40 |
8.148238e+10 |
AIR.PA |
Airbus SE |
0.10 |
0.03 |
0.20 |
7.461750e+10 |
GOOGL |
Alphabet Inc. |
0.10 |
0.03 |
0.30 |
1.183262e+12 |
AMZN |
Amazon.com, Inc. |
0.20 |
0.05 |
0.50 |
1.355935e+12 |
AAPL |
Apple Inc. |
0.15 |
0.00 |
0.40 |
1.765546e+12 |
BA.L |
BAE Systems plc |
0.00 |
-0.05 |
0.05 |
1.787769e+10 |
CSCO |
Cisco Systems, Inc. |
0.00 |
-0.05 |
0.05 |
1.811978e+11 |
BN.PA |
Danone S.A. |
0.00 |
-0.05 |
0.05 |
3.849391e+10 |
FB |
Facebook, Inc. |
0.05 |
-0.05 |
0.05 |
7.102801e+11 |
GE |
General Electric Company |
0.05 |
0.00 |
0.05 |
9.526443e+10 |
HYU.F |
Hyundai Corporation |
0.10 |
0.00 |
0.30 |
3.768063e+10 |
IDR.MC |
Indra Sistemas, S.A. |
0.00 |
-0.05 |
0.02 |
1.320735e+09 |
INTC |
Intel Corporation |
0.00 |
-0.02 |
0.05 |
2.213519e+11 |
IBM |
International Business Machines Corporation |
0.05 |
-0.02 |
0.10 |
1.000500e+11 |
IAG.MC |
International Consolidated Airlines Group, S.A. |
0.50 |
-0.10 |
1.00 |
1.080973e+10 |
PHIA.AS |
Koninklijke Philips N.V. |
0.00 |
-0.05 |
0.05 |
4.355928e+10 |
MEL.MC |
Meliá Hotels International, S.A. |
0.20 |
0.00 |
0.50 |
1.345669e+09 |
MRK |
Merck & Co., Inc. |
0.05 |
0.00 |
0.05 |
1.683183e+11 |
MSFT |
Microsoft Corporation |
0.10 |
0.00 |
0.15 |
1.537831e+12 |
NESN.SW |
Nestlé S.A. |
0.00 |
-0.05 |
0.03 |
2.751414e+11 |
NFLX |
Netflix, Inc. |
0.05 |
-0.01 |
0.15 |
2.033889e+11 |
NHH.MC |
NH Hotel Group, S.A. |
0.20 |
-0.05 |
0.50 |
1.475451e+09 |
NOKIA.HE |
Nokia Corporation |
0.05 |
-0.05 |
0.20 |
1.926819e+10 |
NVDA |
NVIDIA Corporation |
0.10 |
-0.05 |
0.30 |
2.806550e+11 |
ORCL |
Oracle Corporation |
0.05 |
0.00 |
0.05 |
1.641228e+11 |
PEP |
PepsiCo, Inc. |
0.00 |
-0.05 |
0.05 |
1.651222e+11 |
QCOM |
QUALCOMM Incorporated |
0.05 |
-0.02 |
0.15 |
1.291990e+11 |
SSUN.F |
Samsung Electronics Co., Ltd. |
0.10 |
-0.02 |
0.20 |
4.059750e+11 |
SIE.DE |
Siemens Aktiengesellschaft |
0.05 |
-0.02 |
0.10 |
1.086902e+11 |
ENR.DE |
Siemens Energy AG |
0.05 |
-0.02 |
0.10 |
2.160643e+10 |
SPOT |
Spotify Technology S.A. |
0.02 |
-0.05 |
0.10 |
4.376889e+10 |
TSLA |
Tesla, Inc. |
0.00 |
-0.20 |
0.05 |
5.454659e+11 |
TXN |
Texas Instruments Incorporated |
0.05 |
0.00 |
0.10 |
1.416198e+11 |
BA |
The Boeing Company |
0.05 |
-0.05 |
0.10 |
1.208403e+11 |
KHC |
The Kraft Heinz Company |
0.00 |
-0.05 |
0.05 |
4.085665e+10 |
PG |
The Procter & Gamble Company |
0.05 |
-0.05 |
0.05 |
2.802307e+11 |
DIS |
The Walt Disney Company |
0.05 |
-0.05 |
0.20 |
2.940217e+11 |
TMO |
Thermo Fisher Scientific Inc. |
0.05 |
-0.05 |
0.15 |
1.527621e+11 |
TM |
Toyota Motor Corporation |
0.05 |
-0.02 |
0.10 |
1.805378e+11 |
UBER |
Uber Technologies, Inc. |
0.10 |
-0.10 |
0.20 |
8.531728e+10 |
UL |
Unilever PLC |
0.00 |
-0.05 |
0.05 |
1.977436e+11 |
# Get market reference (MSCI All World Accumulative ETF in USD)
market = pd.DataFrame(yf.download(ALL_WORLD, start=START_DATE, end=END_DATE)['Adj Close'])
market.columns = ['Market Ref:USD']
cc = CurrencyConverter(fallback_on_missing_rate=True, fallback_on_wrong_date='True')
for index in market.index:
original_value = market.at[index, 'Market Ref:USD']
value = cc.convert(original_value, 'USD', 'EUR', date=index.to_pydatetime())
market.at[index, 'Market Ref:EUR'] = value
# Fill any NA values with the best forward or backarwd value
market.fillna(method='ffill', inplace=True)
del cc, original_value, value, index
[*********************100%***********************] 1 of 1 completed
# Clean up dataframes and keep only EUR with the symbol name
# Same for Market
for column in stocks.columns:
if ':EUR' not in column:
stocks.drop(column, axis='columns', inplace=True)
symbol, currency = column.split(':')
stocks.rename(mapper={column: symbol}, axis='columns', inplace=True)
for column in market.columns:
if ':EUR' not in column:
market.drop(column, axis='columns', inplace=True)
symbol, currency = column.split(':')
market.rename(mapper={column: symbol}, axis='columns', inplace=True)
del column, symbol, currency
# Create a checkpoint
stocks.to_csv('cache_stocks.csv')
market.to_csv('cache_market.csv')
# Restore Checkpoint
stocks = pd.read_csv('cache_stocks.csv')
stocks.set_index(pd.DatetimeIndex(stocks['Date']), inplace=True)
stocks.drop(['Date'], axis='columns', inplace=True)
market = pd.read_csv('cache_market.csv')
market.set_index(pd.DatetimeIndex(market['Date']), inplace=True)
market.drop(['Date'], axis='columns', inplace=True)
# Construct the dictionary with your views
views = stocks_pd['Prediction'].to_dict()
# Construct a uncertanties dictionary
optimistic = stocks_pd['Optimistic'].to_dict()
pesimistic = stocks_pd['Pesimistic'].to_dict()
variances = []
for symbol in views.keys():
sigma = (optimistic[symbol] - pesimistic[symbol])/2
variances.append(sigma ** 2)
omega = np.diag(variances)
del optimistic, pesimistic, variances, symbol, sigma
# Construct the Black Litterman
S = risk_models.CovarianceShrinkage(stocks).ledoit_wolf()
delta = black_litterman.market_implied_risk_aversion(market['Market Ref'])
# Using a pi equal weighted as the market caps for stocks are too complex to calculate
bl = BlackLittermanModel(S, pi="market", market_caps=stocks_pd['Market Cap'],
risk_aversion=delta, absolute_views=views, omega=omega)
ret_bl = bl.bl_returns()
S_bl = bl.bl_cov()
from pypfopt import EfficientFrontier, objective_functions
ef = EfficientFrontier(ret_bl, S_bl)
ef.add_objective(objective_functions.L2_reg)
ef.max_sharpe()
weights = ef.clean_weights()
pd.Series(weights).plot.pie(figsize=(10,10));
/home/luis/.local/lib/python3.8/site-packages/pypfopt/efficient_frontier.py:195: UserWarning: max_sharpe transforms the optimisation problem so additional objectives may not work as expected.
warnings.warn(
/home/luis/.local/lib/python3.8/site-packages/pandas/plotting/_matplotlib/core.py:1547: MatplotlibDeprecationWarning: normalize=None does not normalize if the sum is less than 1 but this behavior is deprecated since 3.3 until two minor releases later. After the deprecation period the default value will be normalize=True. To prevent normalization pass normalize=False
results = ax.pie(y, labels=blabels, **kwds)
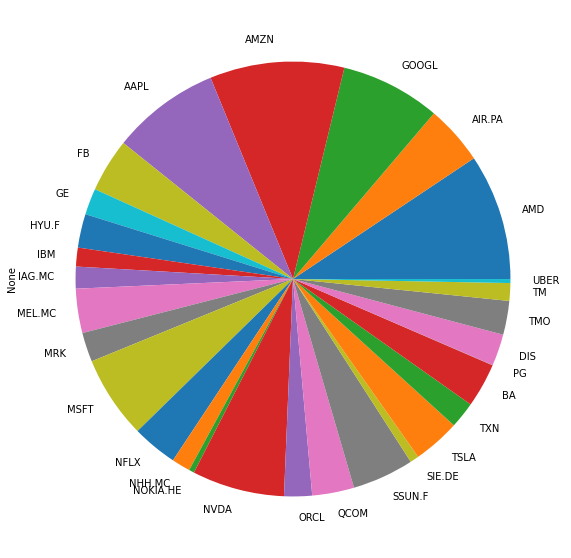
from pypfopt import DiscreteAllocation
da = DiscreteAllocation(weights, stocks.iloc[-1], total_portfolio_value=TOTAL_FUNDS)
alloc, leftover = da.greedy_portfolio()
allocation = pd.DataFrame(data=stocks_pd['Company Name'],
index=stocks_pd.index, columns=['Company Name'])
for key in alloc.keys():
allocation.at[key, 'Desired Position'] = alloc[key]
allocation.at[key, 'Last Price EUR'] = int(stocks.iloc[-1][key] * 100)/100
allocation.at[key, 'Total Funds EUR'] = int(stocks.iloc[-1][key] * alloc[key] * 100)/100
allocation.fillna(0, inplace=True)
allocation['Desired Position'] = allocation['Desired Position'].astype(int)
allocation
|
Company Name |
Desired Position |
Last Price EUR |
Total Funds EUR |
Symbol |
|
|
|
|
AMD |
Advanced Micro Devices, Inc. |
46 |
81.66 |
3756.74 |
AIR.PA |
Airbus SE |
19 |
89.62 |
1702.78 |
GOOGL |
Alphabet Inc. |
2 |
1522.77 |
3045.55 |
AMZN |
Amazon.com, Inc. |
2 |
2713.83 |
5427.66 |
AAPL |
Apple Inc. |
28 |
112.19 |
3141.34 |
BA.L |
BAE Systems plc |
0 |
0.00 |
0.00 |
CSCO |
Cisco Systems, Inc. |
0 |
0.00 |
0.00 |
BN.PA |
Danone S.A. |
0 |
0.00 |
0.00 |
FB |
Facebook, Inc. |
7 |
230.63 |
1614.46 |
GE |
General Electric Company |
80 |
9.66 |
773.20 |
HYU.F |
Hyundai Corporation |
29 |
34.29 |
994.69 |
IDR.MC |
Indra Sistemas, S.A. |
0 |
0.00 |
0.00 |
INTC |
Intel Corporation |
0 |
0.00 |
0.00 |
IBM |
International Business Machines Corporation |
5 |
109.24 |
546.21 |
IAG.MC |
International Consolidated Airlines Group, S.A. |
374 |
1.73 |
649.82 |
PHIA.AS |
Koninklijke Philips N.V. |
0 |
0.00 |
0.00 |
MEL.MC |
Meliá Hotels International, S.A. |
237 |
5.53 |
1311.79 |
MRK |
Merck & Co., Inc. |
12 |
71.47 |
857.75 |
MSFT |
Microsoft Corporation |
13 |
186.91 |
2429.83 |
NESN.SW |
Nestlé S.A. |
0 |
0.00 |
0.00 |
NFLX |
Netflix, Inc. |
3 |
436.74 |
1310.22 |
NHH.MC |
NH Hotel Group, S.A. |
159 |
3.46 |
550.14 |
NOKIA.HE |
Nokia Corporation |
48 |
3.23 |
155.20 |
NVDA |
NVIDIA Corporation |
5 |
457.92 |
2289.63 |
ORCL |
Oracle Corporation |
15 |
54.12 |
811.92 |
PEP |
PepsiCo, Inc. |
0 |
0.00 |
0.00 |
QCOM |
QUALCOMM Incorporated |
9 |
132.99 |
1196.92 |
SSUN.F |
Samsung Electronics Co., Ltd. |
1 |
1382.00 |
1382.00 |
SIE.DE |
Siemens Aktiengesellschaft |
2 |
124.30 |
248.60 |
ENR.DE |
Siemens Energy AG |
0 |
0.00 |
0.00 |
SPOT |
Spotify Technology S.A. |
0 |
0.00 |
0.00 |
TSLA |
Tesla, Inc. |
2 |
700.34 |
1400.68 |
TXN |
Texas Instruments Incorporated |
5 |
143.26 |
716.30 |
BA |
The Boeing Company |
7 |
182.55 |
1277.86 |
KHC |
The Kraft Heinz Company |
0 |
0.00 |
0.00 |
PG |
The Procter & Gamble Company |
0 |
0.00 |
0.00 |
DIS |
The Walt Disney Company |
6 |
153.26 |
919.56 |
TMO |
Thermo Fisher Scientific Inc. |
2 |
429.14 |
858.29 |
TM |
Toyota Motor Corporation |
4 |
131.02 |
524.09 |
UBER |
Uber Technologies, Inc. |
2 |
48.17 |
96.34 |
UL |
Unilever PLC |
0 |
0.00 |
0.00 |
allocation.to_excel('/mnt/wd-bigfoot/share/luis/OneDrive/Documents/Portfolio/99 Last Allocation of Stocks.xlsx',
index=True)